多光谱温度测量反演算法的比较与分析
2025,33(1):293-300
摘要:多光谱测温方法相较于传统单光谱测温方法而言,其具有非接触测量,响应速度快,鲁棒性好等优点,被广泛的用于科学研究,工业生产等领域。然而,光谱发射率的求解是多光谱测温方法中无法避免的问题;通过引入一种参考温度模型可将光谱发射率的求解转换为带约束的极小值优化问题。对于此问题,使用构建发射率模型进行仿真以及利用实际测量数据进行验证的方法,实现了对两种启发式算法和三种其混合算法性能的检验和评估;结果表明,遗传算法(GA)总体精度较高,粒子群算法(PSO)速度较快,三种混合算法在一定程度上平衡了两种算法的性能优势;实际测量数据的反演结果表明,五种算法的平均相对误差均小于1.17%,证明了它们在实际测量中的实用性;对比分析了不同算法的反演发射率和温度性能,为不同场景和优化目标下的算法选择提供依据。
关键词:多光谱温度测量;发射率;参考温度模型;启发式算法;算法分析
Comparison and Analysis of Inversion Algorithms for Multispectral Temperature Measurements
Abstract:Multi-spectral temperature measurement technology is widely used in scientific research, industrial production and other fields due to its non-contact measurement, fast response time, higher accuracy and better robustness compared with single-spectral temperature measurement technology. However, for many targets whose emissivity cannot be measured in advance, the unknown spectral emissivity will directly affect the measurement accuracy. By introducing a reference temperature model, the solution of spectral emissivity can be transformed into a constrained minimum optimization problem. To solve this problem, the performance of two heuristic algorithms and three hybrid algorithms are tested and evaluated by constructing emissivity model and verifying with actual measurement data The simulation results show that the genetic algorithm (GA) has relatively higher overall accuracy, the particle swarm algorithm (PSO) is relatively faster in terms of speed, and the three hybrid algorithms balance the performance advantages of these two algorithms to some extent; The inversion results of the actual measurement data show that the mean relative errors of the five algorithms are less than 1.17%, which proves their practicality in actual measurements. This work compares and analyzes the performance of inversion emissivity and temperature of different algorithm, which provides a basis for algorithm selection under different scenarios and optimization targets.
Key words:Multi-spectral temperature measurement; Emissivity; Reference temperature model; Heuristic algorithm; Algorithm analysis
收稿日期:2024-11-20
基金项目:国家自然科学基金资助项目(62105305);山西省基础研究计划资助项目(20210302123068,202203021212141);山西省高等学校科技创新项目(2022L004);
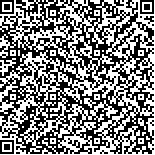