基于改进MFCC特征提取和DNN网络的机器人语音识别方法研究
2025,33(2):246-253
摘要:为了实现机器人语音控制,并避免环境噪音的干扰,研究提出了基于Mel频率倒谱系数特征提取和深度神经网络的机器人语音控制指令识别方法。实验结果显示,相较于其他语音增强方法,基于深度神经网络和谐波增强技术的语音增强方法分段信噪比和语音质量感观评价均更高。同时相比于其他特征,研究提出的基于改进Mel频率倒谱系数特征能显著降低语音识别的字错误率,通过辅以改进深度神经网络-隐马尔科夫模型能进一步降低字错误率。在20dB条件下,该特征和改进深度神经网络-隐马尔科夫模型的平均字错误率分别为24.9%和22.1%,均低于其他方法。上述结果表明,研究提出的语音识别方法能实现带噪声语音的准确识别,提高机器人的语音控制指令识别能力。
关键词:语音识别;语音增强;声学模型;MFCC特征;DNN
Research on Robot Speech Recognition Method Based on Improved MFCC Feature Extraction and DNN NetworkKaixin Qin1 Weixin Wang2 Yansheng Wang3
Abstract:In order to achieve robot voice control and avoid environmental noise interference, a robot voice control instruction recognition method based on Mel frequency cepstral coefficient feature extraction and deep neural network is proposed. The experimental results show that compared to other speech enhancement methods, the speech enhancement method based on deep neural networks and harmonic enhancement technology has higher segmented signal-to-noise ratio and perceived speech quality evaluation. Compared to other features, the improved Mel frequency cepstral coefficient feature proposed in the study can significantly reduce the word error rate in speech recognition, and further reduce the word error rate by combining it with an improved deep neural network hidden Markov model. Under the condition of 20dB, the average word error rates of this feature and the improved deep neural network hidden Markov model are 24.9% and 22.1%, respectively, both lower than other methods. The above results indicate that the proposed speech recognition method can achieve accurate recognition of noisy speech and improve the speech control command recognition ability of robots.
Key words:Speech recognition; Speech enhancement; Acoustic model; MFCC features; DNN
收稿日期:2024-08-29
基金项目:2022年云南省哲学社会科学规划项目(YB2022085);2024年全国教育规划青年课题(EHA210438)
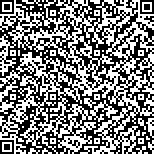