基于改进U-Net网络的双能CT材料分解方法
2025,33(1):252-260
摘要:双能计算机断层扫描(DECT)能够提供扫描对象的定量信息,实现材料分解,进而获取有关人体组织材料特定信息。针对传统的U-Net网络从DECT图像中提取非局部特征受到限制的问题,提出了一种改进的U-Net网络(IU-Net),旨在提高DECT图像材料分解的准确性。IU-Net采用多尺度编码器,在编码阶段,通过三条路径从不同角度捕捉输入图像的局部和非局部特征,并在通道维度上进行融合。同时,为避免过度平滑导致的图像细节损失,引入了边缘损失来构造混合损失函数。优化了重建图像的边缘像素,产生更清晰的图像。实验结果表明,IU-Net结合混合损失函数减少了伪影,同时保留了更多组织细节,胸部骨图的结构相似性达到了0.9967。
关键词:边缘损失函数;神经网络;材料分解;能谱CT;多尺度编码
Dual-Energy CT Material Decomposition based on Improved U-Net Network
Abstract:Dual-energy computed tomography (DECT) can provide quantitative information of scanned objects, decompose materials, and obtain specific information about human tissues and materials. Aiming at the problem that the traditional U-Net network is limited in extracting non-local features from DECT images, an improved U-Net network (IU-Net) was proposed to improve the accuracy of DECT image material decomposition. IU-Net uses a multi-scale encoder. In the encoding stage, the local and non-local features of the input image are captured from different perspectives through three paths and fused in the channel dimension. At the same time, in order to avoid the loss of image details caused by excessive smoothing, the edge loss is introduced to construct a hybrid loss function. The edge pixels of the reconstructed image are optimized to produce a sharper image. Experimental results show that IU-Net combined with the hybrid loss function reduces the artifacts while retaining more tissue details, and the structural similarity of the chest bone map reaches 0.9967.
Key words:edge loss function; neural network;material decomposition; energy spectrum CT;multiscale coding
收稿日期:2024-08-14
基金项目:国家自然科学基金项目(面上项目,重点项目,重大项目)(62201520); 山西省基础研究计划 (202103021224190,202203021222052); 山西省留学基金2023-129
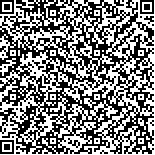