基于DGNN的巨型星座星地跟踪任务规划
2025,33(1):218-225
摘要:随着低轨星座的规模日益扩大,地面测控站所承担的测控任务量也相应增加,这些任务的执行不仅需要与卫星建立星地连接,还需要对卫星进行精确的跟踪;对如何设计高效的规划算法并制定有效的星地连接策略进行了研究;通过对巨型星座网络的特征进行了分析,提出了以可见时间窗为时间分割依据建模网络为离散动态图的方法,然后采用了动态图神经网络(DGNN)的关键技术和方法,表现和处理其复杂的空间动态关系;通过公开的OneWeb星座数据进行模拟仿真,设计实验验证方案的可行性;多个DGNN的实验结果表明,巨型星座的星地跟踪数据的动态变化能够通过DGNN有效的地捕捉和学习到,且该网络在对星地跟踪的未来时间点进行预测时表现出了较高的准确性。
关键词:巨型星座;星地跟踪;任务规划;神经网络;动态图神经网络;
Research on The Planning of Giant Constellation Earth-tracking Mission Based on DGNN
Abstract:With the increasing scale of low-orbit constellation, the number of measurement and control tasks undertaken by ground T C stations increases accordingly. The execution of these tasks requires not only the establishment of satellite-earth connection with satellites, but also the accurate tracking of satellites. How to design an efficient planning algorithm and formulate an effective satellite-earth connection strategy is studied. Based on the analysis of the characteristics of the giant constellation network, a method of discrete dynamic graph based on the visible time window is proposed. Then, the key technology and method of dynamic graph neural network (DGNN) is adopted to represent and deal with its complex spatial dynamic relationship. Through the open OneWeb constellation data simulation, design experiments to verify the feasibility of the scheme. The experimental results of multiple DGNN show that the dynamic changes of the star-earth tracking data of the giant constellation can be effectively captured and learned by DGNN, and the network shows high accuracy in predicting the future time point of star-earth tracking.
Key words:giant constellations; satellite and earth tracking; task planning; neural network; dynamic graph neural network;
收稿日期:2024-07-30
基金项目:
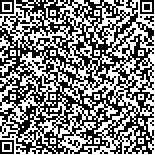