基于MYOLOv8的目标检测方法
2025,33(1):93-98
摘要:针对当前的目标检测方法难以表征多尺度上下文特征的问题,提出了MYOLOv8算法;为了提高YOLOv8模型对于小、中、大型目标的检测能力,提出了一种分层多尺度提取模块对空间特征进行分层特征聚合来捕获多尺度空间上下文信息;为了进一步提高模型对于空间语义的提取能力,提出了一种自适应的通道注意力机制,该机制通过自适应地学习相邻通道之间的相互依赖关系来促进模型关注有用特征,抑制无用特征;为了提高模型对于边界困难样本的定位能力,提出了一种Slide Loss来处理目标检测中的样本不平衡问题,该方法采用对困难样本进行强加权的方式来促使模型着重优化难分样本;在MS COCO数据集上的实验结果表明,所提出的算法相比于YOLOv8-n和YOLOv8-s,mAP分别提升了3.4%和1.4%,同时具有相似的参数量和计算开销,以及更快的推理速度。
关键词:目标检测;多尺度上下文;分层特征聚合;注意力机制;样本不平衡
Object detection method based on MYOLOv8
Abstract:The MYOLOv8 algorithm is proposed to address the problem that the current object detection methods make it hard to represent multi-scale context features. In order to improve the detection capability of YOLOv8 model for small, medium and large objects, a hierarchical multi-scale extraction module is proposed to perform hierarchical feature aggregation of spatial features to capture multi-scale spatial context information. In order to further improve the model's ability to extract spatial semantics, a self-adaptive channel attention mechanism is proposed, which promotes the model to focus on useful features and suppress useless features by adaptively learning the interdependencies between adjacent channels. In order to improve the localization ability of model for boundary difficult samples, a Slide Loss is proposed to deal with the sample imbalance problem in object target detection, which employs a strong weighting on the difficult samples to motivate the model to focus on optimizing the difficult samples. Experimental results on the MS COCO dataset shows that the proposed algorithm improves the mAP by 3.4% and 1.4% compared to YOLOv8-n and YOLOv8-s, respectively, while having similar the number of parameters and computational cost, as well as faster inference speed.
Key words:object detection; multi-scale context; hierarchical feature aggregation; attention mechanism; sample imbalance
收稿日期:2024-07-25
基金项目:西安交通工程学院中青年基金(2022KY-33);陕西省教育厅科学研究计划项目(23JK0529)。
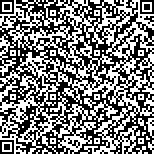