带最大熵修正和GAIL的PPO算法
2025,33(1):235-241
摘要:为提高智能体在策略优化过程中的探索性和稳定性,改善强化学习中智能体陷入局部最优和奖励函数设置问题,提出了一种基于最大熵修正和GAIL的PPO算法;在PPO框架内引入最大熵修正项,通过优化策略熵,鼓励智能体在多个可能的次优策略间进行探索,从而更全面地评估环境并发现更优策略。同时,为解决强化学习过程中因奖励函数设置不合理引起的训练效果不佳问题,引入GAIL思想,通过专家数据指导智能体进行学习;实验表明,引入最大熵修正项和GAIL的PPO算法在强化学习任务上取得了良好的性能,有效提升了学习速度和稳定性,且能有效规避因环境奖励函数设置不合理引起的性能损失。该算法为强化学习领域提供了一种新的解决策略,对于处理具有挑战性的连续控制问题具有重要意义。
关键词:强化学习;PPO算法;生成式对抗模仿学习;深度学习;最大熵学习
PPO Algorithm with Maximum—Entropy Correction and GAIL
Abstract:To enhance the exploration capability and stability of agents during policy optimization, and to address the issues of local optima and reward function setting in reinforcement learning, a PPO algorithm based on maximum entropy correction and GAIL has been proposed. Within the PPO framework, a maximum entropy correction term is introduced, which optimizes the entropy of the policy to encourage the agent to explore among multiple potential suboptimal policies, thereby enabling a more comprehensive assessment of the environment and the discovery of superior strategies. Meanwhile, to tackle the suboptimal training outcomes stemming from ill-conceived reward function settings in reinforcement learning, the concept of GAIL is incorporated, guiding the agent's learning process through expert data. Experimental results demonstrate that the PPO algorithm incorporating maximum entropy correction and GAIL achieves remarkable performance in reinforcement learning tasks, effectively boosting learning speed and stability while effectively mitigating performance degradation caused by ill-suited reward function designs in the environment. This algorithm offers a novel solution in the field of reinforcement learning and holds significant implications for tackling challenging continuous control problems.
Key words:Reinforcement learning; PPO algorithm; Generative adversarial imitation learning; Deep learning; Maximum entropy learning
收稿日期:2024-07-22
基金项目:
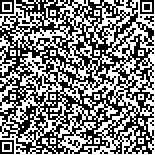