基于改进粒子群优化算法的声音识别及健康检测研究
2024,32(11):87-94
摘要:声音信号作为一种典型的非稳态信号,其复杂性和多样性对信号处理技术提出了更高的要求。研究提出一种基于改进粒子群优化算法的声音识别模型,通过声音实现对新生儿的健康状况分析。该模型通过小波去噪对声音数据进行预处理,然后引入改进的粒子群优化算法与反向传播算法相结合,优化声音识别模型。实验结果表明,在数据集为1000时,小波去噪模型的信噪比为0.97,结构信息损失率为0.18,交并比为0.96。针对不同类型的声音,改进粒子群优化算法模型识别的准确率分别为0.87、0.83、0.97、0.88,均方根误差值为0.09、0.07、0.05、0.07。结果表明,所提出的声音识别与健康检测模型能够有效提高声音数据的识别精度和检测效率,有助于对新生儿健康状态的评估。
关键词:健康检测;声音识别;粒子群优化算法;小波去噪;反向传播
Research on Sound Recognition and Health Detection Based on Improved Particle Swarm Optimization Algorithm
Abstract:The crying of newborns can to some extent reflect their health status. Healthy newborns may cry loudly, while unhealthy newborns may cry weakly and weakly. However, as a typical non-stationary signal, the complexity and diversity of sound signals place higher demands on signal processing techniques. Therefore, a sound recognition model based on improved particle swarm optimization algorithm was proposed to analyze the health status of newborns through sound. The model preprocesses the sound data through wavelet denoising, and then combines the improved particle swarm optimization algorithm with backpropagation algorithm to optimize the sound recognition model. The experimental results show that when the dataset is 1000, the signal-to-noise ratio of the wavelet denoising model is 0.97, the structural information loss rate is 0.18, and the intersection to union ratio is 0.96. The accuracy of the improved particle swarm optimization algorithm model for different types of crying sounds is 0.87, 0.83, 0.97, and 0.88, with RMSE values of 0.09, 0.07, 0.05, and 0.07, respectively. The experimental results show that the proposed voice recognition and health detection model can effectively improve the recognition accuracy and detection efficiency of sound data, and evaluate the health status of newborns.
Key words:Health testing; Voice recognition; Particle swarm optimization algorithm; Wavelet denoising; Back propagation
收稿日期:2024-07-20
基金项目:辽宁省科技计划联合计划(应用基础研究项目):复杂环境下智能生产线工况识别及故障诊断关键技术研究(2023JH2/101700066)
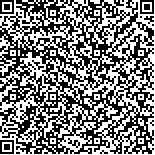