基于BOA-DF-LightGBM的入侵检测方法
2024,32(12):88-95
摘要:入侵检测模型在训练时经常面临数据不平衡问题,即其中正常行为的样本数量远远超过异常入侵行为的样本数量;为解决数据不平衡问题,将深度森林和LightGBM结合作为入侵检测模型,其中通过深度森林中的多粒度扫描生成更丰富的特征作为LightGBM的输入,从而提升分类器的性能。并且深度森林生成的特征表示可以提高少数类样本的可分性,配合 LightGBM 的权重调整机制,可以更好地处理不平衡数据问题,并通过全局搜索能力强大的棕熊优化算法对模型进行参数调优进一步提升模型的预测准确度;经UNSW_NB15数据集验证所提方法,BOA-DF-LightGBM模型较其他模型指标更为优异,预测准确率达到95.15%,较DF提升了近2%;为进一步验证其对数据不平衡问题的能力,通过更严苛的数据不平衡实验得出,BOA-DF-LightGBM模型在数据不平衡实验中的准确率为94.23%,较DF提升了2.68%,较神经网络模型提升了3.42%;验证了BOA-DF-LightGBM在数据不平衡情况下的有效性和优异性。
关键词:入侵检测;集成学习;棕熊优化算法;深度森林
Intrusion detection method based on BOA-DF-LightGBM
Abstract:Intrusion detection models often face the problem of data imbalance during training, that is, the number of samples of normal behavior far exceeds the number of samples of abnormal intrusion behavior. In order to solve the problem of data imbalance, the deep forest and LightGBM are combined as an intrusion detection model, in which richer features are generated by multi-granularity scanning in the deep forest as the input of LightGBM, so as to improve the performance of the classifier. Moreover, the feature representation generated by deep forest can improve the distinguishability of minority samples, and with the weight adjustment mechanism of LightGBM, it can better deal with unbalanced data problems, and the brown bear optimization algorithm with powerful global search ability is used to tune the parameters of the model to further improve the prediction accuracy of the model. The proposed method is verified on the UNSW_NB15 dataset, and the BOA-DF-LightGBM model is better than other model indicators, with the prediction accuracy reaching 95.15%, which is nearly 2% higher than DF. In order to further verify its ability to solve the problem of data imbalance, the accuracy of the BOA-DF-LightGBM model in the data imbalance experiment is 94.23%, which is 2.68% higher than that of DF and 3.42% higher than that of neural network model. The effectiveness and superiority of BOA-DF-LightGBM in the case of data imbalance are verified.
Key words:Intrusion detection; Ensemble learning; Brown Bear Optimization algorithm;Deep Forest;
收稿日期:2024-06-28
基金项目:温州市科研项目(ZF2022003)
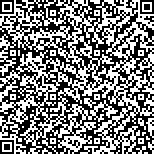