城市排水管道缺陷智能检测方法研究
2024,32(12):81-87
摘要:针对城市排水管道缺陷自动化检测准确率低、目标定位不准确的问题,提出一种改进YOLOv8的排水管道缺陷检测模型。该模型在基线模型中引入感受野注意力卷积,并构建了C2F_RFAConv模块,通过空间感受野与卷积交互自适应学习的方式,增强模型对缺陷特征的提取能力;同时,提出一种混合注意力高低阶特征融合网络,将Backbone和Neck输出的三种不同尺度的低阶特征和高阶特征进行有效融合,增强模型学习图像全局上下文信息的能力;此外,对影响边界框回归的重叠情况、中心点距离、宽高偏差等因素进行了综合分析,设计Inner-MPDIoU损失函数,使模型适应不同尺寸的缺陷检测任务,提高缺陷目标边界框的定位准确率。经过实验验证发现,改进后的模型取得了93.9%的平均检测准确率,较改进之前提升3.7%,漏检率和误检率仅为9.1%和17.6%,较改进之前分别降低3.2%和2.7%。
关键词:城市排水管道;缺陷检测;YOLOv8;感受野空间注意力;特征融合;Inner-MPDIoU
Research on intelligent detection method of defects in urban drainage pipelines
Abstract:To address the problems of low automatic detection accuracy and inaccurate target positioning of urban drainage pipeline defects, an improved YOLOv8 drainage pipeline defect detection model is proposed. This model introduces receptive field attention convolution into the baseline model and constructs the C2F_RFAConv module to enhance the model's ability to extract defect features through interactive adaptive learning of spatial receptive fields and convolutions. Additionally, a hybrid attention high-order and-low-order feature fusion network is proposed, which effectively fuses the low-order and high-order features of three different scales output by the backbone and neck, enhancing the model's ability to learn the global contextual information of the image. The Inner-MPDIoU loss function is designed by comprehensively analyzing factors affecting bounding box regression, such as overlap, center point distance, and width-height deviation. This function enables the model to adapt to defect detection tasks of different sizes and improves the positioning accuracy of the defect target boundary box. Experimental validation shows that the improved model achieves an average detection accuracy of 93.9%, which is a 3.7% increase compared to the baseline model; the missed detection rate and false detection rate are reduced to 9.1% and 17.6%, representing decreases of 3.2% and 2.7%, respectively, compared to the baseline model.
Key words:Urban drainage pipes; Defect detection; YOLOv8; RFAConv; Feature fusion;Inner-MPDIoU
收稿日期:2024-05-30
基金项目:陕西省自然科学基金面上项目(2024JC-YBMS-286)
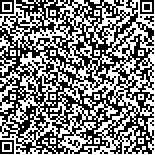