基于YOLOv5架构改进的电磁信号目标识别方法
2024,32(11):243-250
摘要:针对从在时间及频段上重叠的多种电磁信号中识别出目标信号的问题,提出了一种基于YOLOv5架构改进的电磁信号目标识别方法。通过改进 YOLOv5算法以提高对电磁信号时频图像上目标信号的检测精度。采用MobileNetV3作为主干网络,以实现骨干网络轻量化的同时平衡精度和速度;通过在特征金字塔网络模块中引入自适应空间特征融合(ASFF)模块来将不同尺度的特征进行融合,调整损失函数,从而增强网络对不同尺度目标的感知能力。将改进YOLOv5算法在自制电磁信号时频图像数据集上与原始YOLOv5算法和SSD算法进行对照试验。实验表明,改进后的方法对时频图像上目标信号的检测能力有明显增强,具有一定的实用价值。
关键词:深度学习;目标检测;电磁信号识别;自适应空间特征融合;MobileNet
An Improved Electromagnetic Signal Target Recognition Method Based on the YOLOv5 Architecture
Abstract:In addressing the problem of identifying target signals from multiple overlapping electromagnetic signals in both time and frequency domains, a method based on an improved YOLOv5 architecture for electromagnetic signal target recognition is proposed. By enhancing the YOLOv5 algorithm, the detection accuracy of target signals in time-frequency images of electromagnetic signals is improved. MobileNetV3 is used as the backbone network to achieve a balance between precision and speed while ensuring the backbone network remains lightweight. The adaptive spatial feature fusion (ASFF) module is introduced into the feature pyramid network module to merge features of different scales and adjust the loss function, thereby enhancing the network's ability to perceive targets of various scales. The improved YOLOv5 algorithm is compared with the original YOLOv5 algorithm and the SSD algorithm on a custom electromagnetic signal time-frequency image dataset. Experiments show that the improved method significantly enhances the detection capability of target signals in time-frequency images, demonstrating practical value.
Key words:Deep Learning ; Target Detection; Electromagnetic Signal Recognition; Adaptive Spatial Feature Fusion; MobileNet
收稿日期:2024-05-17
基金项目:江苏省重点研发项目(BE2022065-5)
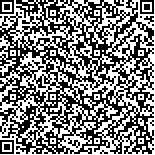