基于GAN的红外小目标检测合成数据增强技术研究
2024,32(11):118-124
摘要:近年来,卷积神经网络(CNNs)在红外小目标检测方面取得了良好的效果。然而,有限的公共训练数据限制了基于CNN方法的性能提升。为了解决训练数据的稀缺问题,提出了一种生成红外小目标检测合成训练数据的方法。采用生成对抗网络框架,其中合成背景图像和红外小目标在两个独立的过程中生成。在第一阶段,通过将可见光图像转化为红外图像来合成红外图像。在第二阶段,将转换后的图像上植入目标掩码。然后,所提出的强度调制网络合成了真实的目标对象,可以从进一步的图像处理产生。在最新的公共数据集上的实验结果表明,当使用由真实图像和合成图像组成的数据集训练各种检测网络时,检测网络比只使用真实数据产生更好的性能。
关键词:卷积神经网络(CNN);生成对抗网络(GAN);图像转换;红外小目标;合成数据增强
GAN-Based Synthetic Data Augmentation for Infrared Small Target Detection Technology Research
Abstract:Recently, convolutional neural networks (CNNs) have achieved state-of-the-art performance in infrared small target detection. However, the limited number of public training data restricts the performance improvement of CNN-based methods. To handle the scarcity of training data, we propose a method that can generate synthetic training data for infrared small target detection. We adopt the generative adversarial network framework where synthetic background images and infrared small targets are generated in two independent processes. In the first stage, we synthesize infrared images by transforming visible images into infrared ones. In the second stage, target masks are implanted on the transformed images. Then, the proposed intensity modulation network synthesizes realistic target objects that can be diversely generated from further image processing. Experimental results on the recent public dataset show that, when we train various detection networks using the dataset composed of both real and synthetic images, detection networks yield better performance than using real data only.
Key words:convolutional neural network (CNN); generative adversarial network (GAN); image translation; infrared small target; synthetic data augmentation.
收稿日期:2024-04-30
基金项目:2023年江苏省产学研合作项目(BY20231025)。
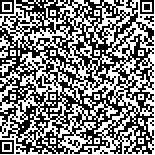