基于BEV视角的多传感融合3D目标检测
2024,32(10):77-85
摘要:3D目标检测是自动驾驶在道路环境感知任务中的重要环节,现有主流框架通过搭载多种感知设备获取多模态的数据信息来实现多传感融合检测;传统相机与激光雷达的传感器融合过程中存在几何失真,以及信息优先级不对等,导致传感融合的3D目标检测性能不足;对此,提出了一种基于鸟瞰视角(BEV)的多传感融合3D目标检测算法;利用提升-展开-投射(LSS)方式,获取图像的潜在深度分布建立图像在BEV空间下的特征;采用PV-RCNN的集合抽象法建立点云在BEV空间下的特征;该算法在统一的BEV共享空间中设计了低复杂度的特征编码网络融合多模态特征实现3D目标检测;实验结果表明,所提出的算法在检测精度上相较于纯激光方法提升4.8%,相较于传统的融合方案减少了47%的参数,并保持了相近的精度,较好地满足了自动驾驶系统道路环境感知任务的检测要求。
关键词:3D目标检测;鸟瞰图视角;多传感融合;自动驾驶;道路环境感知
Multi-sensor Fusion 3D Object Detection Based on BEV Perspective
Abstract:3D object detection is an important part of the road environment perception task of autonomous driving. Its mainstream framework is a detection solution that uses multiple sensing devices to obtain multi-modal data to achieve multi-sensor fusion. The geometric distortions and unequal information priorities in the traditional sensor fusion process result in insufficient 3D object detection performance of sensor fusion. A multi-sensor fusion 3D object detection algorithm is proposed based on bird's-eye view (BEV). The lift-splat-shot (LSS) method obtains the potential depth distribution of the image and establishes the feature map of the image in the BEV space. The set abstraction method of PV-RCNN is used to establish the feature map of the point cloud in BEV space. A low-complexity feature encoding network is designed for fusing multi-modal features in a unified BEV space in the proposed method to achieve 3D object detection. Experimental results show that the proposed method improves accuracy by 4.8% compared to the pure LiDAR methods, reduces parameters by 47% compared to the traditional fusion methods, and maintains similar accuracy. The proposed method meets the detection requirements of the road environment perception task of the autonomous driving system.
Key words:3D object detection; BEV view; multi-sensor fusion; autonomous driving; road environment perception
收稿日期:2024-04-30
基金项目:国家自然科学基金项目(面上项目,重点项目,重大项目)
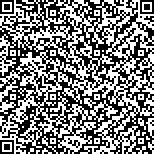