基于改进蜣螂算法的多区域空调系统需求响应DMPC供冷策略
2024,32(10):250-262
摘要:针对夏季电网高峰期间办公建筑空调系统的峰值负荷造成电网短缺等问题,提出一种基于需求响应的多区域空调系统分布式模型预测控制(DMPC)供冷策略;以西安市某办公建筑的5个区域为研究对象,分别建立该办公建筑的物理模型及空调系统能耗数学模型,并验证模型的准确性;构建多区域空调系统仿真模型,优化目标为最小化空调系统运行能耗和室温与设定值的误差最小;选取蜣螂算法作为优化工具,并针对该算法存在全局搜索速度慢、易早收敛和陷入局部最优等缺点;采取混沌映射策略优化种群初始化,生成更加均匀的种群以提升种群个体质量;利用螺旋搜索策略对蜣螂的觅食和繁殖行为进行更新,进一步扩展算法的全局搜索性;同时引入随机扰动和自适应因子改进蜣螂的偷窃行为,改善算法易陷入局部最优等问题。运用改进后的蜣螂算法对DMPC的滚动优化进行优化求解,并与PID温度反馈控制进行对比,验证DMPC的控制性能。实验结果表明,在所研究的5个区域中,DMPC比PID控制方法的响应速度分别提升了8.91%、8.65%、12.04%、5.79%和1.79%。结合需求响应策略利用分时电价进行调控,提出温度与启停优化调控策略对空调系统的峰值负荷进行削峰转移。结果表明两种预冷启停优化策略的峰时负荷转移率分别为27.29%和29.16%,可以有效地将系统高峰时段的冷负荷转移到其他时段,降低电网运行压力。
关键词:需求响应;削峰转移;分布式模型预测控制;改进的蜣螂优化算法;节能优化
Improved Dung Beetle Algorithm-Based Demand Response Cooling Strategy for Multi-Zone Air Conditioning Systems Using Distributed Model Predictive Control
Abstract:To address the issue of peak load demands on power grids during summer caused by office building air conditioning systems, a demand response-based Distributed Model Predictive Control (DMPC) cooling strategy for multi-zone systems was proposed. The study used a physical model and a mathematical model of energy consumption for the air conditioning systems in five zones of an office building in Xi"an, validating their accuracy. The multi-zone air conditioning system simulation aimed to minimize operational energy consumption and the temperature deviation from set points. The dung beetle algorithm was selected for optimization, overcoming its slow global search, premature convergence, and susceptibility to local optima through chaotic mapping for population initialization, helical search strategies for foraging and breeding behaviors, and random perturbations with adaptive factors to improve exploratory behavior. Enhanced with these modifications, the dung beetle algorithm improved the rolling optimization of DMPC, which outperformed PID temperature feedback control, increasing response speeds by 8.91%, 8.65%, 12.04%, 5.79%, and 1.79% in the respective zones. Additionally, demand response strategies incorporating time-of-use electricity rates suggested temperature and start-stop optimization strategies to shift peak loads, achieving peak load transfer rates of 27.29% and 29.16% with the precooling start-stop strategies, effectively redistributing peak cooling loads to off-peak periods and alleviating pressure on the power grid.
Key words:demand response; peak shaving or load shifting; distributed model predictive control; improved dung beetle optimization algorithm; energy saving optimization
收稿日期:2024-04-26
基金项目:国家自然科学基金项目(52278125),陕西省自然科学基础研究基金(2022JM-283),陕西省建设厅科技计划发展项目(2020-K17)
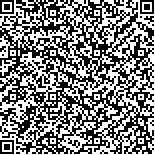