基于混合自注意力孪生网络的小样本轴承故障诊断
2025,33(2):1-8
摘要:针对小样本条件下传统应用于滚动轴承故障诊断的深度学习算法诊断精度不高,泛化性差的问题。提出了一种基于混合自注意力机制和孪生网络的小样本轴承故障诊断算法。基于孪生神经网络结构,构造相同类别和不同类别的轴承样本对输入孪生网络,借助一维卷积单元提取样本的特征,然后采用位置自注意力机制和通道自注意力机制进行矩阵融合构建混合自注意力模块,提取更具判别性的特征信息,距离度量阶段以自适应的网络度量方式来代替常用的欧式距离度量,并且引入全局均值池化来减少网络参数。实验结果表明,本文方法在每类故障样本数量为9、15、30的准确率为88.2%、94.7%、96.2%,对比其他方法,本文所提算法在小样本条件下的诊断准确率更高,并且拥有更好的泛化性能。
关键词:滚动轴承;孪生神经网络;故障诊断;自注意力机制;混合自注意力
Fault Diagnosis of Small Sample Bearing Based on Hybrid Self-Attention Siamese Network
Abstract:Aiming at the problems of poor diagnostic accuracy and poor generalization of the traditional deep learning algorithm applied to rolling bearing fault diagnosis under small sample conditions. A small-sample bearing fault diagnosis algorithm based on hybrid self-attention mechanism and siamese network is proposed. Based on the structure of the siamese neural network, pairs of bearing samples of the same category and different categories are constructed and fed into the siamese network, and the features of the samples are extracted with the help of one-dimensional convolutional unit, and then the hybrid self-attention module is constructed by using the matrix fusion of the positional self-attention mechanism and the channel self-attention mechanism to obtain more discriminative feature information, and the distance metric is used to replace the commonly used Euclidean distance measure with the adaptive network measure, and the global mean distance measure is introduced to replace the commonly used European distance metric. metric, and global mean pooling is introduced to reduce network parameters. The experimental results show that the accuracy of this paper's method is 88.2%, 94.7%, and 96.2% for the number of fault samples of 9, 15, and 30 in each class, comparing with other methods, the algorithm proposed in this paper has a higher diagnostic accuracy under the condition of small samples and has a better generalization performance.
Key words:rolling bearing; siamese neural network; fault diagnosis; self-attention mechanism; hybrid self-attention
收稿日期:2023-11-26
基金项目:贵州省科技计划项目(黔科合战略找矿[2022]ZD001))
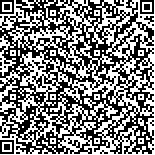