FEV-YOLOv8n:轻量化安全帽佩戴检测方法
2025,33(1):69-77
摘要:针对基线YOLOv8n检测算法结构较复杂以及现有的安全帽佩戴检测算法参数量和计算量较大,难以在终端部署等问题,提出一种基于FEV-YOLOv8n的轻量化检测模型。设计一种轻量级的FasterC2f模块改进YOLOv8n的骨干网络,实现网络的参数量和计算量的降低;在FasterC2f模块中引入EMA注意力机制,融合空间依赖和位置信息,建立长短期的依赖关系,增强对目标表征的关注,以提高模型检测的精度;使用VoVGSCSP模块改进颈部网络,提高遮挡目标以及小目标的辨识度;实验结果表明,改进YOLOv8n模型map值为92.5%,相较于YOLOv8n算法,模型大小减少20%,计算量降低18.5%,参数量降低15.7%,为安全帽佩戴检测的轻量化研究提供理论参考。
关键词:目标检测;安全帽;FasterC2f;轻量化;Efficient Multi-Scale Attention;VoVGSCSP
FMV-YOLOv8n:Lightweight Helmet Wearing Detection Methods
Abstract:Aiming at the problems that the structure of baseline YOLOv8n detection algorithm is more complicated and the existing helmet wearing detection algorithm has a large number of parameters and computation, which is difficult to be deployed at the terminal, a lightweight detection model based on FEV-YOLOv8n is proposed. A lightweight FasterC2f module is designed to improve the backbone network of YOLOv8n, realizing the reduction of the number of parameters and computation of the network; the EMA attention mechanism is introduced into the FasterC2f module, fusing spatial dependence and positional information, establishing long and short-term dependence relationships, and enhancing the attention to the target's representation, in order to improve the accuracy of the model's detection; and VoVGSCSP is used to improve the neck network, improving the accuracy of the helmet wearing detection algorithm. module to improve the neck network to improve the recognition of occluded targets as well as small targets; the experimental results show that the map value of the improved YOLOv8n model is 92.5%, which reduces the model size by 20%, computation by 18.5%, and parameter count by 15.7% compared to the YOLOv8n algorithm, which provides theoretical references for the study of the lightweighting of helmet wearing detection.
Key words:target detection; Safety Helmet; FasterC2f; lightweighting; Efficient Multi-Scale Attention; VoVGSCSP
收稿日期:2023-11-17
基金项目:新疆维吾尔自治区自然科学基金资助项目(2022D01A202);新疆维吾尔自治区高校科研计划项目(XJEDU2020Y020)
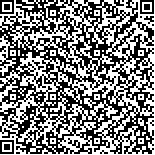