自适应多点最优最小熵反褶积在风电齿轮箱轴承故障诊断中的应用
2024,32(11):34-40
摘要:齿轮箱在风力发电机组传动系统中起着重要的作用,因此齿轮箱故障诊断是风力发电机组健康管理中的一个关键问题。考虑到齿轮箱振动信号的频谱复杂性,多点最小最优熵反褶积方法是一种简单有效的齿轮箱故障诊断方法,因为它不仅可以去除掉大量的背景噪声和振动干扰,与此同时还能突出微弱的轴承故障脉冲信号。但是该方法的性能在一定程度上取决于前置参数滤波器长度的选择,不合适的滤波器参数值可能会导致过滤不足或过度过滤的后果。为了解决这一问题,提出了一种基于樽海鞘优化算法的自适应最优最小熵反褶积方法,该方法可以自适应选择最优滤波器长度,从而达到最优滤波效果。最后,利用包络解调方法对最优滤波信号进行包络分析得到包络谱,从而揭示故障特征频率。通过对某风力发电机实验台齿轮箱信号的仿真和实验分析,说明了该方法的原理和有效性。
关键词:风电机组;齿轮箱;故障诊断;樽海鞘优化算法;最小最优熵反褶积
Application of Adaptive Multipoint Optimal Minimum Entropy Deconvolution for Bearing Fault Diagnosis of Wind Turbine Gearboxes
Abstract:The gearbox plays a crucial role in the transmission system of wind turbines, making gearbox fault diagnosis a pivotal issue in wind turbine health management. Considering the spectral complexity of gearbox vibration signals, multipoint optimal minimum entropy deconvolution emerges as a straightforward and effective approach for gearbox fault diagnosis. This method not only eliminates interference such as background noise and vibration interferences but also highlights weak fault-induced? impulse signals. The performance of this method, however, is contingent upon the appropriate selection of the pre-parameter of filter length to a certain extent. Inadequate or excessive filtering may result from an inappropriate choice. In order to address this issue, an adaptive optimal minimum entropy deconvolution method based on the salp swarm algorithm is proposed in this paper, which enables the adaptive selection of the optimal filter length and achieves superior filtering efficacy. The envelope spectrum of the optimal filtered signal is ultimately obtained through the utilization of the envelope demodulation technique, thereby revealing the fault characteristic frequency. The principle and effectiveness of the proposed method are demonstrated through the simulation and experimental analysis of the gearbox signal extracted from a wind turbine test stand.
Key words:wind turbine; gearbox; fault diagnosis; salp swarm algorithm; multipoint optimal minimum entropy deconvolution
收稿日期:2023-10-07
基金项目:西京学院科研基金资助项目(XJ220206)
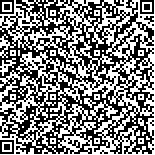