基于LSTM神经网络的烟丝水分恒定控制系统设计
2024,32(11):177-183
摘要:在烟丝加工过程中,水分分布受到温度、湿度多个因素的影响,控制系统无法准确反映整体水分情况。为全面提高加工型香烟的质量水平,设计基于LSTM神经网络的烟丝水分恒定控制系统。部署Profibus控制总线,并在线路体系中连接水分检测仪与水分恒定器,完成烟丝水分恒定控制系统的硬件设计。在系统软件设计方面,构建LSTM神经网络单元,根据烟叶吸湿能力分析条件,求解具体的水分分布模型,实现基于LSTM神经网络的烟丝水分模型建模。分别计算烟叶出口湿度与出口温度,并联合传递函数逼近参量与恒定时滞参数,完成对控制参数的整定处理,再联合相关应用部件,实现基于LSTM神经网络的烟丝水分恒定控制系统设计。实验结果表明,LSTM神经网络模型作用下,生丝含水量被稳定控制在13%-18%数值之间,不会因水分过量问题而导致香烟质量水平无法达到实际加工标准。
关键词:LSTM神经网络;烟丝水分;恒定控制;Profibus总线;吸湿能力;水分模型;出口湿度;出口温度;
Design of tobacco moisture constant control system based on LSTM neural network
Abstract:In the process of tobacco processing, the distribution of moisture is influenced by multiple factors such as temperature and humidity, and the control system cannot accurately reflect the overall moisture situation. To comprehensively improve the quality level of processed cigarettes, a tobacco moisture constant control system based on LSTM neural network is designed. Deploy the Profibus control bus and connect the moisture detector and moisture constant device in the circuit system to complete the hardware design of the tobacco moisture constant control system. In terms of system software design, an LSTM neural network unit is constructed, and based on the analysis conditions of tobacco moisture absorption capacity, a specific moisture distribution model is solved to achieve the modeling of tobacco moisture model based on LSTM neural network. Calculate the outlet humidity and outlet temperature of tobacco leaves separately, and combine the transfer function approximation parameters and constant time delay parameters to complete the tuning process of control parameters. Then, combine relevant application components to achieve the design of a tobacco moisture constant control system based on LSTM neural network. The experimental results show that under the action of the LSTM neural network model, the moisture content of raw silk is stably controlled between 13% -18%, and the problem of excessive moisture will not cause the quality level of cigarettes to fail to meet the actual processing standards.
Key words:LSTM neural network; Tobacco moisture; Constant control; Profibus Bus; Hygroscopic ability; Water model; Outlet humidity; Outlet temperature;
收稿日期:2023-09-20
基金项目:
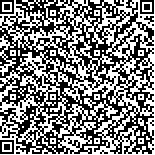