精密摆组件装配设备自标定方法
2023,31(7):228-233
摘要:精密装配设备中各运动滑台存在安装误差,影响装配精度;目前,多采用装配设备内部集成的显微视觉系统,借助特制标定板进行线性标定;但该方法中的误差参数顺序标定,解算具有关联性,无法避免误差传递与累积的问题,且精密装配设备空间有限,标定板一般较难安装;为此,基于精密摆组件装配设备,提出一种通用自标定方法,该方法不借助标定板,仅需要在装配作业空间中由视觉系统跟踪设备自身固定特征的若干任意点位,再结合粒子群优化(Particle Swarm Optimization, PSO)完成误差参数的统一求解,最后根据推导的误差补偿模型实现误差补偿;实验证明,该方法与线性标定相比精度及稳定性均明显提高,标定精度在5 μm以内,为精密装配设备提供了简单有效的标定方案。
关键词:微装配;显微视觉;自标定;粒子群优化;误差补偿
Self-calibration Method of Precision Pendulum Assembly Equipment
Abstract:There are installation errors in each sliding table in precision assembly equipment, which affect the assembly accuracy; At present, the microscopic vision system integrated in the assembly equipment is mostly used for linear calibration with the help of a special calibration board; However, the error parameters in this method are sequentially calibrated and the solution is correlated, which can not avoid the problems of error transmission and accumulation, and the space of precision assembly equipment is limited, so the calibration board is generally difficult to install; Therefore, based on the precision pendulum assembly equipment, a general self-calibration method is proposed. This method does not use calibration board and only requires the visual system to track several arbitrary points of fixed features of the equipment itself in the assembly workspace, and then combine with particle swarm optimization (PSO) to complete the unified solution of the error parameters, and finally realize error compensation based on the derived error compensation model. The image coordinates in the compensation model correspond to the motion pulse of the sliding table one by one, and the mapping from the geometric domain to the control domain is realized. Experiments show that the accuracy and stability of this method are obviously improved compared with linear calibration, and the calibration accuracy is less than 5 μm, which provides a simple and effective calibration scheme for precision assembly equipment.
Key words:micro-assembly; microscopic vision; self-calibration; particle swarm optimization; error compensation
收稿日期:2023-05-11
基金项目:辽宁省“兴辽英才计划”资助项目(No.XLYC2002020);辽宁省自然科学(编号:2020-MS-104)
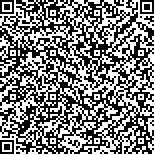