改进蜜獾算法优化OTSU的图像分割研究
2023,31(9):260-266
摘要:针对图像分割算法存在分割效果不理想的缺点, 采用反向学习策略和柯西变异因子对蜜獾算法进行改进, 进而结合二维OTSU用于图像阈值分割;首先, 通过反向学习策略改进蜜獾种群的初始化, 提高种群多样性和分布平衡, 从而提高算法的整体搜索能力;其次, 引入柯西变异因子, 对算法计算得到的可行解进行扰动, 使算法更易于跳出局部最优, 增强算法的局部搜索能力和收敛精度;最后, 通过MHBA优化后的二维OTSU对3幅标准图像进行分割验证;实验证明, MHBA-OTSU算法得到的分割图像精度更高、效果更细致, 验证了方法的有效性。
关键词:二维OTSU算法;蜜獾算法;反向学习策略;柯西变异;图像分割
Improved Honey Badger Algorithm to Optimize OTSU for Image Segmentation
Abstract:To address the shortcomings of image segmentation algorithms with unsatisfactory segmentation effects, this paper improves the honey badger algorithm through the backward learning strategy and the Corsi variation factor, and then combines the two-dimensional OTSU for image threshold segmentation. First, the initialization of the honey badger population is improved by the reverse learning strategy to enhance the population diversity and distribution balance, thus improving the overall search ability of the algorithm; second, the Corsi variation factor is introduced to perturb the feasible solutions calculated by the algorithm, making it easier for the algorithm to jump out of the local optimum and enhancing the local search ability and convergence accuracy of the algorithm; finally, the two-dimensional OTSU optimized by MHBA is used for the segmentation of three Finally, the segmentation of the standard images is verified by the MHBA-optimized 2D OTSU. The experiment proves that the segmented images obtained by MHBA-OTSU algorithm have higher accuracy and more detailed effect, which verifies the effectiveness of the method.
Key words:two-dimensional OTSU algorithm; Honey badger algorithm; Reverse learning strategy; Cauchy mutation; image segmentation
收稿日期:2023-04-02
基金项目:国家自然科学基金项目(62172249),国家自然科学基金项目(61973180)
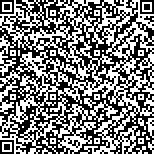