带状态约束的事件触发积分强化学习控制
2023,31(7):143-149
摘要:为克服全状态对称约束以及控制策略频繁更新的局限,同时使得无限时间的代价函数最优,针对一类具有部分动力学未知的仿射非线性连续系统,提出一种带状态约束的事件触发积分强化学习的控制器设计方法。该方法是一种基于数据的在线策略迭代方法。引入系统转换将带有全状态约束的系统转化为不含约束的系统。基于事件触发机制以及积分强化学习算法,通过交替执行系统转换、策略评估、策略改进,最终系统在满足全状态约束的情况下,代价函数以及控制策略将分别收敛于最优值,并能降低控制策略的更新频率。此外,通过构建李亚普诺夫函数对系统以及评论神经网络权重误差的稳定性进行严格的分析。单连杆机械臂的仿真实验也进一步说明算法的可行性。
关键词:仿射非线性系统;最优控制;事件触发控制;积分强化学习;神经网络
Event-Triggered Integral Reinforcement Learning Optimal Control with state constraints
Abstract:In order to overcome the limitations of the full-state symmetry constraints and the frequent update of the control policy, and to make the infinite horizon cost function optimal, a controller design method with event-triggered integral reinforcement learning with state constraints is proposed for a class of affine nonlinear continuous systems with partial unknown dynamics. It is a data-based online policy iteration approach. Firstly, system transformation is introduced to transform a constrained system into an unconstrained system. Next, based on the event triggering mechanism and integral reinforcement learning algorithm, by alternating system transformation, policy evaluation, and policy improvement, the system will satisfy the full-state constraints, the cost function and control policy will converge make optimal. At the same time, it can reduce the update frequency of the control policy. In addition, the stability of the system is strictly analyzed by constructing the Lyapunov function. The simulation experiment of the single-link robotic arm is given to verify the effectiveness of the proposed approach.
Key words:affine nonlinear system; optimal control; event-triggering control; integral reinforcement learning; neural network
收稿日期:2023-02-24
基金项目:国家自然科学基金(No.61833007)
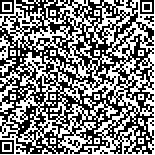