基于WOA-XGBoost模型的网络入侵检测
2023,31(3):127-133
摘要:网络入侵检测系统(NIDS)是检测网络攻击和维护网络安全的关键技术之一,是网络安全领域中的重要研究方向。近年来,研究者利用机器学习算法来完成入侵检测任务并取得了很好的成果,但检测效率和精确率有待进一步提升。在对鲸鱼优化算法(WOA)和极限梯度提升算法(XGBoost)的特点进行实验和对比分析的基础上,提出了WOA-XGBoost模型,首先构建基于XGBoost的分类模型,然后利用WOA算法自适应搜索XGBoost的最优参数,最后基于NSL-KDD数据集评估所提出WOA-XGBoost模型的性能。实验结果表明,该模型在分类精确率、准确率、召回率和AP指标方面均优于其他模型如XGBoost、随机森林、Adaboost和LightGBM。该工作也为群体智能优化算法在网络入侵检测中的应用提供了依据。
关键词:网络安全;入侵检测;异常行为检测;WOA-XGBoost;集成学习
Network Intrusion Detection Based on WOA-XGBoost Model
Abstract:Network intrusion detection system (NIDS) is one of the key technologies to detect network attacks and protect network security, and it is an important research direction in the field of network security. In recent years, researchers have used machine learning algorithms to complete intrusion detection tasks and achieved good results, but the detection efficiency and accuracy need to be further improved. Based on the experiments and comparative analysis of the characteristics of the whale optimization algorithm (WOA) and the extreme gradient boosting algorithm (XGBoost), the WOA-XGBoost model is proposed. First, a classification model based on XGBoost is constructed, and then the optimal parameters of XGBoost are searched adaptively using the WOA algorithm. Finally evaluate the performance of the proposed WOA-XGBoost model based on the NSL-KDD dataset. Experimental results show that the model outperforms other models such as XGBoost, Random Forest, Adaboost and LightGBM in terms of classification precision, accuracy, recall and AP indicators. This work also provides a basis for the application of swarm intelligence optimization algorithm in network intrusion detection.
Key words:cyber security; intrusion detection; abnormal detection; WOA-XGBoost; ensemble learning
收稿日期:2023-01-19
基金项目:
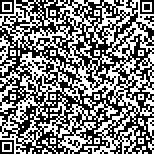