基于TSNS-RAE的多模态过程故障检测
2023,31(9):22-28
摘要:根据多模态工业生产过程的数据特点,提出基于时空近邻标准化和鲁棒自编码器(TSNS-RAE)的故障检测方法;TSNS处理数据时同时考虑了样本的时间近邻和空间近邻,可以消除数据动态性和多模态特征;相比于普通的自编码器,鲁棒自编码器提升了模型的抗噪性和鲁棒性,具有更好的提取非线性特征的能力;TSNS-RAE模型将原始数据空间分成模型空间和残差空间两部分,选择残差空间的SPE统计量作为监控统计量,通过数值案例和青霉素实验来验证 TSNS-RAE的可行性。
关键词:多模态;故障检测;鲁棒自编码器;时空近邻标准化; 青霉素生产过程
Multi-modal process fault detection based on Time-Space Nearest Neighborhood Standardization and Robust AutoEncoder
Abstract:Aiming at the characteristics of multimodal industrial processes, A fault detection method based on Time-Space Nearest Neighborhood Standardization and Robust AutoEncoder (TSNS-RAE) is proposed. TSNS processes data by considering both temporal and spatial neighbors of samples, thus eliminating data dynamics and multimodal features; Compared with ordinary autoencoders, robust autoencoders improve the noise resistance and robustness of the model, and have better ability to extract nonlinear features. The TSNS-RAE model divides the original data space into model space and residual space, and selects SPE statistics of residual space as monitoring statistics. Numerical cases and penicillin experiments are used to verify the feasibility of TSNS-RAE.
Key words:multimode; Fault detection; Robust AutoEncoder; Time-Space Nearest Neighborhood Standardization
收稿日期:2022-12-01
基金项目:国家自然科学基金资助项目、(61490701,61673279);辽宁省教育厅重点实验室项目(LJ2020021)
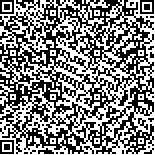