模型未知LTI系统的数据驱动预测控制
2023,31(9):116-123
摘要:针对含有随机噪声的模型未知线性时不变 (Linear Time Invariant, LTI) 系统模型建立过程复杂且控制律难以得到的问题,提出一种基于数据驱动的预测控制方法。基于系统行为学理论和平衡子系统辨识方法,仅利用测量得到的系统数据构建被控系统的非参数模型,将其和预测控制理论相结合设计出基于数据驱动的预测控制器,对于系统测量数据中存在的有界加性高斯噪声,通过引入数据的松弛变量和L2正则项来降低噪声扰动的影响,采用滚动时域优化策略计算最优控制序列并将其作用于被控系统,实现系统对设定值的轨迹跟踪。将所提控制策略应用于四容水箱系统,仿真结果表明与同样基于数据驱动的子空间预测控制方案相比,所提方法具有更好的动态性能,且该策略在抗噪声扰动方面有明显优势,具有更强的鲁棒性。
关键词:数据驱动;系统行为学理论;线性时不变系统;预测控制;滚动时域优化;轨迹跟踪;高斯噪声
Data-driven Predictive Control for Model-unknown Linear Time Invariant system
Abstract:To solve the problem that the modeling process of model unknown Linear Time Invariant (LTI) system containing stochastic noise is complicated and the control law is difficult to be obtained, a data-driven predictive control method is proposed. Based on the theory of system behavior and balanced subspace identification, the non-parametric model of the controlled system is built only by using the measured system data, and the data-driven predictive controller is designed by combining it with the predictive control theory. For the bounded additive gaussian noise existing in the measured data, the influence of noise disturbance is reduced by introducing the slack variable and quadratic regularization of the data. The receding horizon optimization strategy is used to calculate the optimal control trajectory and apply it to the controlled system to realize the stability control of the system. The proposed control strategy is applied to a quadruple tank system. The simulation results show that compared with the data-driven subspace predictive control scheme, the pr\posed strategy has better dynamic performance. Meanwhile, the proposed strategy also has obvious advantages in anti-noise disturbance and stronger robustness.
Key words:Data-driven; Behaviour System Theory; linear time invariant system; predictive control; receding horizon optimization; trajectory tracking; gaussian noise
收稿日期:2022-11-29
基金项目:国家自然科学(62073154)
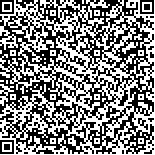