基于滑动窗口的直升机序列异常检测算法
2023,31(2):41-47
摘要:无标签的序列在异常检测算法中往往存在着对数据的信息掌握不全面、不能合理使用的情况,而采用深度学习的技术实现检测时往往对其计算的解释性欠佳;对于攻克这些难题,以直升机飞行数据为例对时间序列的反常检测问题展开了深入研究,并利用Iforest技术和PCA算法,给出了一个采用滑动窗口的时间序列异常检测方法,利用从滑动窗口采集信息的时间变化状态等数据信息,将序列异常检测问题转换为点异常检测问题;同时以auc评分为衡量标准,从带有时刻特殊标志的多个信息集上检验了检测效率的提高;在无标签的直升机飞行数据集上进行实验,验证了算法的有效性,并通过对比检测过程中不同特征变量的变化情况,从算法层面和现实层面上阐述了算法的可解释性。
关键词:异常检测;序列异常;点异常;直升机飞行数据
An Algorithm for Helicopter Sequence Anomaly Detection Based on Sliding Window
Abstract:In the unlabeled sequence anomaly detection problem, the acquisition of data sequence features is not sufficient and cannot be effectively used, and the deep learning method is often used to detect the algorithm has poor interpretation. In order to solve the above problems, the helicopter flight data was taken as an example to study the anomaly detection of time series. Based on Iforest algorithm and PCA algorithm, a sliding window based sequence anomaly detection algorithm was proposed. By extracting the fluctuation and statistical information of the data through the sliding window, the sequence anomaly detection problem was transformed into a point anomaly detection problem. auc score was selected as the measurement standard to verify the improvement of the detection efficiency of the algorithm on multiple data sets with abnormal labels. Experiments were carried out on the unlabeled helicopter flight data set to verify the effectiveness of the algorithm. By comparing the changes of different characteristic variables in the detection process, the interpretability of the algorithm was illustrated from the algorithm level and the practical level.
Key words:anomaly detection; abnormal sequence; anomalies;helicopter flight data
收稿日期:2022-11-16
基金项目:
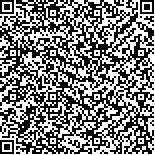