融合YOLO v3与改进ReXNet的手势识别方法研究
2023,31(7):278-283
摘要:工程应用中的手势识别需要较高的实时性和准确性,而现场环境通常无法提供足够的计算能力,采用轻量化神经网络在解决了上述问题的同时,还能达到与深度神经网络相当的识别效果。为此,提出一种基于改进轻量化神经网络的手势识别方法。该方法改进用于手部关键点检测的ReXNet网络结构,以改善骨骼点的局部关注;同时将关键点检测损失函数MSE替换为Huber loss,以提升离群点的抗干扰性。实验环境搭建基于普通单目镜头捕获图像后,经YOLO v3手部识别模型和改进的ReXNet关键点检测模型,并根据约束手部骨骼关键点的向量角而定义的不同手势,最后达到实时检测的效果。改进模型在RWTH公开数据集上的测试结果表明,改进后的手势识别方法的检测准确度较改进前整体提升2.62%,达到了96.18%,且收敛速度更快。
关键词:手势识别;关键点检测;YOLO v3;ReXNet ;Ghost Module
Research on Gesture Recognition Method Integrating YOLO v3 and Improved ReXNet
Abstract:Gesture recognition in engineering applications requires high real-time and accuracy, and the on-site environment usually cannot provide sufficient computing power. Using lightweight neural networks can solve the above problems while achieving recognition equivalent to deep neural networks Effect. To this end, a gesture recognition method based on an improved lightweight neural network is proposed. This method improves the ReXNet network structure for hand key point detection to improve the local attention of bone points; at the same time, the key point detection loss function MSE is replaced by Huber loss to improve the anti-interference of outliers. After the experimental environment is built based on the ordinary monocular lens to capture the image, the YOLO v3 hand recognition model and the improved ReXNet key point detection model are used to define different gestures according to the vector angles that constrain the key points of the hand bones, and finally achieve real-time detection. Effect. The test results of the improved model on the RWTH public data set show that the detection accuracy of the improved gesture recognition method is 2.62% higher than that before the improvement, reaching 96.18%, and the convergence speed is faster.
Key words:gesture recognition; key point detection; YOLO v3; ReXNet; Ghost Module
收稿日期:2022-10-22
基金项目:国家自然科学基金青年基金资助项目(61903183)
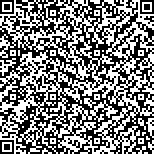