基于深度学习的智能化高精度测向方法
2023,31(1):15-21
摘要:提出了一种基于深度学习的智能化高精度快速波达方向(DOA)估计算法,根据神经网络通过数据驱动而不依赖阵列流型的特点,设计了基于卷积神经网络的PhaseDOA-Net回归网络模型实现估计算法,引入特定模块对输入信号进行特征提取和处理,提高网络模型的拟合效果,用所提网络模型自主学习相位差矩阵与DOA之间的映射关系;引入残差网络结构,解决了卷积神经网络层数加深导致网络退化的问题;仿真生成了具有噪声与幅相误差的信号数据集,并构建信号相位差矩阵作为输入;仿真结果表明,本算法可以提供更高精度的估计性能,大幅减小了估计时间,解决了现有方法在阵列模型误差条件下无法准确得到DOA结果的问题;通过基于实际信号环境中采集数据的训练与测试,验证了系统对不同噪声、幅相误差的鲁棒性以及对不同信号频率更好的适应能力。
关键词:DOA估计;深度学习;神经网络;信号处理;人工智能技术;
Intelligent high accuracy direction finding method based on deep learning
Abstract:An intelligent high accuracy fast direction of arrival(DOA)estimation algorithm based on deep learning is proposed. According to the characteristics of neural network driven by data and independent of array flow pattern,the PhaseDOA-Net regression network model based on convolutional neural network(CNN)is designed, and the residual network structure is introduced to solve the problem of network degradation caused by layer deepening of CNN.Specific modules are designed to extract and process the festures of the input signals,which improves the fitting effect of the networt model.The proposed network model is used to autonomously learn the mapping relationship between the phase difference matrix and DOA.The residual network structure is introduced to solve the problem of network degradation caused by layer deepening of CNN.The data sets with noise and amplitued-phase errors is generated by simulation,and the signal phase difference matrix is constructed as network input.The simulation results show that the algorithm can provide higher accuracy estimation performance, greatly reduce the estimation time, and solve the problems of the existing methods which cannot accurately obtain DOA results under the condition of array model error.Through the training and testing based on the collected data in the actual signal environment,the robustness of the system to different noises,amplitude-phase errors and the great adaptability to different signal frequencies are verified.
Key words:DOA estimation; deep learning; neural network; signal processing; aitificial intellegence technology
收稿日期:2022-10-13
基金项目:国家自然科学基金(U19B2028)
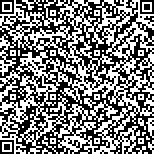