基于深度Q网络和人工势场的移动机器人路径规划研究
2022,30(11):226-232
摘要:随着移动机器人在各个领域的研究与发展,人们对移动机器人路径规划的能力提出了更高的要求;为了解决传统的深度Q网络算法在未知环境下,应用于自主移动机器人路径规划时存在的收敛速度慢、训练前期产生较大迭代空间、迭代的次数多等问题,在传统DQN算法初始化Q值时,加入人工势场法的引力势场来协助初始化环境先验信息,进而可以引导移动机器人向目标点运动,来减少算法在最初几轮探索中形成的大批无效迭代,进而减少迭代次数,加快收敛速度;在栅格地图环境中应用pytorch框架验证加入初始引力势场的改进DQN算法路径规划效果;仿真实验结果表明,改进算法能在产生较小的迭代空间且较少的迭代次数后,快速有效地规划出一条从起点到目标点的最优路径。
关键词:路径规划;DQN;人工势场;栅格地图;pytorch
Research on Path Planning of Mobile Robot Based on Deep Q-Network and Artificial Potential Field
Abstract:With the research and development of mobile robots in various fields, people put forward higher requirements for the ability of mobile robot path planning. So as to solve the problems of slow convergence speed, many iterations, and large iteration space in the early stage of training when the traditional deep reinforcement learning algorithm is applied to the path planning of mobile robots in an unknown environment, an artificial potential field is added when the traditional DQN algorithm initializes the Q value. The attractive field of the algorithm is used as the prior information of the initial environment, so as to guide the mobile robot to move towards the target position, reduce a large number of invalid iterations caused by the environmental exploration in the initial stage of the algorithm, thereby reducing the number of iterations and speeding up the convergence speed. Using the pytorch framework in the grid map environment to verify the path planning effect of the improved DQN algorithm with the initial gravitational potential field. The simulation results show that the improved algorithm can quickly and effectively plan an optimal path from the starting point to the target point after generating a smaller iteration space and fewer iterations.
Key words:path planning;DQN; artificial potential field;grid map; pytorch
收稿日期:2022-09-14
基金项目:国家级大创项目(G014)
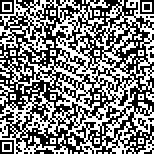