基于局部对比度和全卷积网络的小空间碎片检测
2023,31(7):15-20
摘要:近年来环绕地球轨道的空间碎片数量急剧增加,对在轨运行的航天器构成严重威胁。基于天基监视平台的空间碎片小目标快速检测对于航天器远距离规避具有重要意义,然而由于空间碎片小目标的快速相对运动和宇宙射线产生的噪声,空间碎片小目标快速检测极具挑战。提出了一种基于深度卷积神经网络的天基空间碎片小目标显著性检测方法,首先,使用局部对比度方法获得输入图像的空间对比度图;然后,通过全卷积网络结合上述对比度图捕获时空显著性信息;最后,基于仿真视频序列图像开展实验验证,可实现对最远距离为30km,最小为16像素的目标准确检测。通过设置不同的高斯白噪声方差参数模拟空间环境噪声,证明了在噪声背景条件下对空间碎片小目标检测的有效性和鲁棒性。
关键词:空间碎片;小目标;局部对比度;显著性检测;卷积神经网络
Small Space Debris Detection via Local Contrast Measure and Fully Convolutional Network
Abstract:The drastic increase of the number of space debris in orbit around the earth poses a serious threat to operational spacecraft. Rapid small space debris detection with space-based surveillance platform is very important for spacecraft emergency avoidance at distance. Nevertheless, small space debris detection is a great challenge due to its fast movement and noise caused by cosmic rays in space-based surveillance platform. Inspired by strong pattern recognition capacity of deep learning, a deep convolutional neural network based small space debris saliency detection method for space-based surveillance system is proposed. First, the spatial contrast map of the input image is obtained using local contrast method. Then, the spatiotemporal saliency information is captured incorporating with the above contrast map. Last, the experiments are conducted based on the synthetic video sequence images. It can detect the space debris with the furthest distance 30 km and the smallest size of 16 pixels. The experimental results prove the applicability and robustness of the proposed method through different Gaussian white noise variance set up simulating the cosmic noise.
Key words:space debris; small target; local contrast measure; saliency detection; convolutional neural network
收稿日期:2022-09-12
基金项目:中央高校基本科研业务费(NP2019105)南京航空航天大学博士学位论文创新与创优基金(BCXJ19-11)
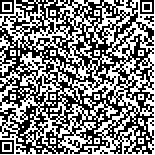