基于频域特征变分自编码器的轴承故障诊断研究
2023,31(4):70-75
摘要:针对深度学习故障诊断模型泛化能力差、网络复杂的问题,提出一种通用的特征提取网络,在此基础上应用轴承故障诊断的方法。首次提出频域特征变分自编码器,增强了信号特征提取的鲁棒性。然后,采用局部异常因子算法剔除离群点,防止分类器过拟合,提高分类器泛化性能。最后,构建分类器进行故障诊断。实验验证表明在不同损伤程度下特征提取的界限清晰,故障分类效果好,并且模型表现出良好的可迁移性。
关键词:故障诊断;特征提取;频域特征;变分自编码器;离群点检测
Research on Bearing Fault Diagnosis Based on Frequency Domain Characteristic Variational Autoencoder
Abstract:Aiming at the problems of poor generalization ability and complex network of deep learning fault diagnosis model, a general feature extraction network is proposed, and the method of bearing fault diagnosis is applied on this basis. The frequency domain feature variational autoencoder is proposed for the first time, which enhances the robustness of signal feature extraction. Then, a local outlier algorithm is used to eliminate outliers, prevent the classifier from overfitting, and improve the generalization performance of the classifier. Finally, a classifier is constructed for fault diagnosis. The experimental verification shows that the boundary of feature extraction is clear under different damage degrees, the fault classification effect is good, and the model shows good transferability.
Key words:fault diagnosis; feature extraction; frequency domain feature; variational autoencoder; outlier detection
收稿日期:2022-09-08
基金项目:
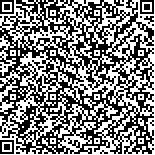