基于对抗的一致性正则半监督辐射源个体识别方法
2023,31(2):204-209
摘要:针对在有标签信号样本数量较少,待识别信号因复杂信道导致数据分布发生变化的实际场景中,传统通信辐射源个体识别技术识别率较低的问题,提出基于对抗的一致性正则半监督辐射源个体识别方法。该方法在一致性正则半监督模型上首次引入基于对抗的域适应思想,建立网络模型提取“域不变”特征,即不同信噪比条件下的信号数据的特征对齐,从而实现在原始信号上训练的模型,对其他信噪比下信号的高准确率识别。在ORACLE射频指纹数据集上通过设置不同条件的数据集展开实验,实验结果表明,基于对抗的一致性正则半监督模型比全监督方法以及经典的一致性正则半监督模型具有更高的识别准确率。
关键词:半监督学习;迁移学习;域适应;辐射源个体识别;射频指纹
Adversarial-based Consistency Regularization Semi-supervised Emitter Identification Methods
Abstract:Aiming at the problem of low recognition accuracy in the actual environment where the number of labeled signal samples is small and the distribution of the signals to be identified is changing due to the actual channel, the adversarial-based consistency egularization semi-supervised emitter identification method was proposed. The method introduces the concept of adversarial-based domain adaptation in the consistency egularization semi-supervised method firstly, and establishes a network model to extract "domain-invariant" features, i.e., the alignment of signal features under different signal-to-noise conditions, so that the improved semi-supervised model trained on the original signal can achieve high accuracy recognition of signals under other signal-to-noise ratios. Experiments are conducted on the ORACLE RF fingerprint dataset with different training set settings, and the experimental results show that the adversarial-based consistency egularization semi-supervised emitter identification method has a higher recognition accuracy than the fully-supervised method and the onsistency egularization semi-supervised method in the actual scenario.
Key words:semi-supervised; transfer learning; domain adaptation; emitter identification; RF fingerprinting
收稿日期:2022-06-23
基金项目:
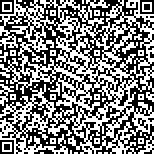