基于ZYNQ的深度学习卷积神经网络加速平台设计
2022,30(12):264-269
摘要:针对将各种卷积神经网络(CNN)模型部署在不同硬件端来实现算法加速时所遇到的耗费时间,工作量大等问题,采用Tengine工具链这一新兴的深度学习编译器技术来设计通用深度学习加速器,来将卷积神经网络模型与硬件后端高效快速对接;深度学习加速器的平台采用ZYNQ系列的ZCU104开发板,采用软硬件协同设计的思想,将开源的英伟达深度学习加速器(NVDLA)映射到可编程逻辑门阵列(FPGA)上,与ARM处理器构成SoC系统;NVDLA整体架构规范,包含软硬件设计,采用Tengine工具链代替原来官方的编译工具链;之后在搭建好的NVDLA平台上实现lenet-5和resnet-18的网络加速,完成了mnist和cifar-10的数据集图像分类任务;实验结果表明,采用Tengine工具链要比NVDLA官方的编译工具链推理速度快2.5倍,并且量化工具使用方便,网络模型部署高效。
关键词:深度学习编译器;NVDLA;卷积神经网络;FPGA;硬件加速
Design of NVDLA Acceleration Platform Based on ZYNQ
Abstract:In view of the timing-consuming and heavy workload problems that encountered when various convolutional neural network (CNN) models are deployed on different hardware to achieve algorithm acceleration, using the Tengine tool chain , an emerging deep learning compiler technology, to design a general deep learning accelerator that can efficiently and fastly connecting the network model and hardware backend. The deep learning accelerator’s platform was a ZYNQ’s ZCU104 development board, the idea of software and hardware co-design was used, the open source Nvidia Deep Learning Acceleator (NVDLA) is mapped on Field Programmable Gate Array (FPGA), and the SoC system was formed with ARM processor. NVDLA’s architecture is very standard, including software and hardware design, the Tengine tool chain is used to replace the original official compilation tool chain. After that, the network of lenet-5 and resnet-18 was realized on the built NVDLA platform, and the image classification task of the mnist and cifar-10 datasets was completed. Experimental results show that the Tengine toolchain is 2.5 times faster than NVDLA’s official compilation toolchain inference speed, and the quantitative tools are easy to use, and the network model deployment is efficient.
Key words:deep learning compiler; NVDLA; convolution neural network; FPGA; hardware acceleration
收稿日期:2022-05-16
基金项目:国家自然科学(51971086);黑龙江省博士后科研启动基金(LBH-Q16118);黑龙江省高校基础研究基金(LGYC2018JC004)
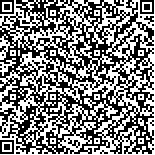