基于CNN-LSTM-PSO的私有云故障检测
2022,30(8):76-82
摘要:有效对私有云系统进行故障检测对于保障IT系统稳定性及开展可靠性信息活动具有重要的实际意义。为此从私有云系统的历史趋势数据出发,将卷积网络(CNN)和长短期记忆(LSTM)循环神经网络结合,提出了基于粒子群优化算法(PSO)的CNN-LSTM-PSO的混合模型,实现对私有云的故障检测。采用X11算法等技术对数据进行预处理,使用CNN网络提取监控指标时序数据的相关特征信息,并通过训练LSTM网络参数建立CNN-LSTM预测模型,设计了PSO算法对预测模型进行参数选优,减小预测误差,并以高斯正态分布确定阈值范围,实现故障的精准检测。通过和传统单一预测模型以及现有的一些组合预测模型的对比,CNN-LSTM-PSO模型预测后结果的均方根误差、平均绝对误差和平均百分比误差都低于其余模型。实验结果验证了模型在预测效果上具备更高的精度和更快的预测速度,在私有云的故障检测中精确性和实时性都具有良好效果。
关键词:LSTM;故障检测;X11分解法;CNN神经网络;PSO算法;高斯正态分布;超参选优
LSTM Private cloud fault detection based on CNN-LSTM-PSO
Abstract:Effective fault detection of private cloud systems is of great practical significance to ensure the stability of IT systems and to carry out reliability information activities. To this end, starting from the historical trend data of private cloud systems, a hybrid model of CNN-LSTM-PSO based on particle swarm optimization (PSO) is proposed by combining convolutional network (CNN) and long short-term memory (LSTM) recurrent neural network., to realize fault detection of private cloud. The X11 algorithm and other technologies are used to preprocess the data, the CNN network is used to extract the relevant feature information of the time series data of the monitoring indicators, and the CNN-LSTM prediction model is established by training the LSTM network parameters, and the PSO algorithm is designed to optimize the parameters of the prediction model and reduce the Small prediction error, and the threshold range is determined by Gaussian normal distribution to achieve accurate fault detection. Compared with the traditional single prediction model and some existing combined prediction models, the root mean square error, mean absolute error and mean percentage error of the predicted results of the CNN-LSTM-PSO model are lower than those of the other models. The experimental results verify that the model has higher accuracy and faster prediction speed in prediction effect, and has good results in both accuracy and real-time performance in fault detection of private cloud.
Key words:LSTM; fault prediction; X11 decomposition method; CNN neural network; PSO algorithm; gaussian normal distribution; multigrid search
收稿日期:2022-03-31
基金项目:国家重点研发计划(2021YFB2900800);上海市科委项目(20511102400)、(20ZR1420900);高等学校学科创新引智计划(111)(D20031)。
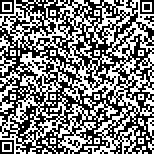