用于PCB缺陷检测的增强上下文信息Yolov4_tiny算法
2022,30(9):80-86
摘要:针对现有的PCB缺陷检测存在检测精度低、速率慢等问题,提出一种用于PCB缺陷检测的增强上下文信息Yolov4_tiny算法。该算法首先通过Transformer编码单元对特征提取网络深层特征冗余的问题进行优化,增强网络捕获不同尺度局部特征信息的能力;然后利用浅层特征增强PCB缺陷小目标上下文信息,提升FPN网络对小目标缺陷的表征能力;最后引入注意力机制对特征提取网络输出的有效特征层加权,强化目标特征表征能力。实验结果表明,该算法对于整体缺陷的平均检测精度的均值(mAP)达到98.70%,较Yolov4_tiny提升了3.12%,实现了PCB缺陷精准定位和识别,满足工业检测的实际需求。
关键词:PCB缺陷检测;Yolov4_tiny;Transformer;上下文信息;注意力机制;
Enhanced Context Information Yolov4_ tinyAlgorithm for PCB Defect Detection
Abstract:Aiming at the problems of low detection accuracy and slow speed in PCB defect detection, an enhanced context information Yolov4_ tiny algorithm for PCB defect detection is proposed. Firstly, the deep feature redundancy of feature extraction network is optimized by Transformer coding unit to enhance the ability of network to capture local feature information at different scales. Then, the shallow features are used to enhance the small target context information of PCB defects and improve the representation ability of FPN network for small target defects. Finally, attention mechanism is introduced to weight the effective feature layer of feature extraction network output to strengthen the ability of target feature representation. The experimental results show that the mean average precision (mAP) of the algorithm for overall defects is 98.70%, which is higher than Yolov4_ tiny increased by 3.12%, realized accurate positioning and identification of PCB defects, and met the actual needs of industrial testing.
Key words:PCB defect detection; Yolov4_ tiny; Transformer; context information; attention mechanism
收稿日期:2022-03-11
基金项目:国家自然科学基金(51678470),陕西省自然科学基础研究计划(2020JM472, 2020JM473, 2019JQ760)
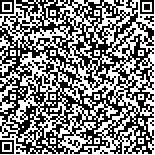