基于深度残差网络的人体行为识别算法研究
2022,30(3):251-258
摘要:针对原始C3D卷积神经网络的层数较少、参数量较大和难以关注关键帧而导致的人体行为识别准确率较低的问题,提出一种基于改进型C3D的注意力残差网络模型;首先,增加原始网络卷积层并采用卷积核合并与拆分操作实现(3x1x7)和(3x7x1)的非对称式卷积核,之后采用全预激活式残差网络结构来增加构建的非对称卷积层,并且在残差块中增加时空通道注意力模块;最后,为展示该算法的先进性和应用性,则将该算法与原始C3D网络以及其他流行算法分别在基准数据集HMDB51和自建的43类别体育运动数据集上相比较;实验结果表明,该算法与原始C3D网络相比,在HMDB51和43类体育运动数据集上分别提高了9.88%和21.61%,参数量比原来降低了38.68%,并且结果也优于其他流行算法。
关键词:深度学习;三维卷积;非对称式卷积核;残差网络;注意力模块;人体行为识别
Research on Human Action Recognition Algorithm Based on Deep Residual Network
Abstract:Aiming at the problem that the original C3D convolutional neural network has a small number of layers, a large amount of parameters, and the difficulty of focusing on key frames lead to the low accuracy of human behavior recognition, an improved C3D-based attention residual network model is proposed. First, add the original network convolution layer and use the convolution kernel merge and split operation to realize the asymmetric convolution kernel of (3x1x7)and(3x7x1), and then the fully pre-activated residual network structure is used to increase the constructed asymmetric convolutional layer, and the spatiotemporal channel attention module is added to the residual block. Finally, in order to demonstrate the advancement and applicability of the algorithm, the algorithm is compared with the original C3D network and other popular algorithms on the benchmark data set HMDB51 and the self-built 43 categories sports data set. Experimental results show that compared with the original C3D network, the algorithm has increased by 9.88% and 21.61% on the HMDB51 and 43 types of sports data sets, respectively, and the amount of parameters has been reduced by 38.68%, and the results are better than other popular algorithms.
Key words:deep learning; three-dimensional convolution; asymmetric convolution kernel; residual network; attention module; human behavior recognition
收稿日期:2022-01-18
基金项目:国家自然科学基金资助项目(60875025)。
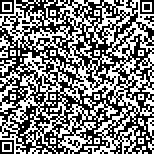