改进FasterRCNN模型的布氏硬度检测方法
2022,30(6):72-78
摘要:自动提取布氏压痕轮廓是提高布氏硬度检测效率的关键一步,针对传统机器视觉算法提取布氏压痕轮廓算法的不足,本文通过FasterRCNN模型实现了布氏硬度压痕直径的自动化检测。针对检测布氏硬度压痕圆的特点,提出对FasterRCNN模型的改进。在classification网络中的边框回归损失函数中加入预测检测框的长与宽的方差,在改进的边框回归函数优化目标修改为真实检测框与预测检测框差距最小且预测检测框宽与高之间差距最小,使得基于改进的FasterRCNN模型布氏硬度检测能够提供更加准确的目标预测检测框,取得更精准检测效果。同时引入数据增强的方法扩充有效数据大小。实验结果表明,基于FasterRCNN的布氏硬度模型检测方法适用于锈蚀和光滑金属表面工况。改进的FasterRCNN网络模型准确率为97.08%,较原模型提升0.73%,归一化均方误差(nMSE)为0.001226,较原模型降低40.31%, 改进的效果明显,并弥补机器视觉算法提取压痕轮廓的不足。
关键词:FasterRCNN;布氏硬度;边框回归;压痕提取;计算机视觉
Measuring Brinell hardness by improving FasterRCNN model
Abstract:Autommatic extraction of Brinell indentation contour is a key step to improve the efficiency of Brinell hardness detection.In order to solve the shortcomings of extracting Brinell indentation contour by traditional machine vision algorithm, an automatic detection of brinell hardness indentation diameter is realized by FasterRCNN model. According to the characteristics of Brinell hardness indentation circle detection, an improved FasterRCNN model is proposed.The variance of length and width of the predicted detection frame is added into the frame regression loss function in the Classification network, and the optimization objective of the improved frame regression function is modified whose goal is to minimize both the gap between the real detection frame and the predicted detection frame and the gap between the width and height of the predicted detection frame. The brinell hardness test based on the improved FasterRCNN model can provide more accurate target prediction detection frame and achieve more accurate detection effect.At the same time, the data enhancement method is introduced to expand the effective data size. The result shows that the Brinell hardness model detection method based on FasterRCNN is suitable for corroded and smooth metal surfaces.Also,the accuracy of the improved FasterRCNN network model is 97.08%, which is 0.73% higher than the original model, and the normalized mean square error (nMSE) is 0.001226, which is 40.31% lower than the original model.The effect on improvement is obvious,and make up for the difficiency of particle swarm dynamic contour model (Snake model).
Key words:FasterRCNN; brinell hardness; box regression; Indentation extraction;machine vision
收稿日期:2021-12-15
基金项目:
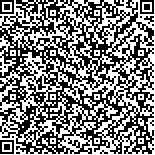