基于DDPG深度强化学习的电站脱硝过程优化控制
2022,30(10):132-139
摘要:针对选择性催化还原(selective catalytic reduction,SCR)脱硝系统脱硝过程存在非线性、多工况等复杂特点,提出一种基于MiniBatchKMeans聚类与Stacking模型融合的SCR脱硝过程NOx预测方法。该方法通过应用MiniBatchKMeans聚类算法对训练集进行工况聚类与划分优化,建立基于XGBoost、随机森林、LightGBM以及线性回归的Stacking融合框架预测模型(Stacking-XRLL),实现电站SCR系统多变工况下NOx排放的精准预测。以广东某电站SCR系统脱硝过程中NOx排放数据为例进行建模仿真与实验,结果表明与单一建模方法多层前馈神经网络(BP)、长短期记忆神经网络(LSTM)以及门控循环单元神经网络(GRU)相比,Stacking-XRLL建模方法的平均预测精确度达到了99%,并最终结合建立好的深度确定性策略梯度(DDPG)强化学习模型,实现电站SCR脱硝过程的参数优化控制。
关键词:多工况;MiniBatchKMeans聚类;Stacking-XRLL;DDPG算法;优化控制
Optimal control of denitrification processes in coal-fired power plants based on deterministic policy gradients with deep reinforcement learning
Abstract:A method for NOx prediction in SCR denitration based on the fusion of MiniBatchKMeans clustering and stacking model is proposed to address the complex characteristics of the denitration process of selective catalytic reduction (SCR) denitration system, such as non-linearity and multiple working conditions.. The method applies the MiniBatchKMeans clustering algorithm to the training set for work condition clustering and partitioning optimization, and establishes the stacking fusion framework prediction model (Stacking-XRLL) based on XGBoost, Random Forest, LightGBM and linear regression to achieve accurate NOx emission prediction under multi-variable work conditions in power station SCR systems. The modeling simulations and experiments were carried out with NOx emission data from the denitrification process of a power station SCR system in China. The results show that the Stacking-XRLL modeling method achieves an average prediction accuracy of 99% compared to the single modeling methods of the multilayer back propagation neural network(BP), long-short term memory neural network(LSTM) and gate recurrent unit neural network(GRU). The final combination of the established deep deterministic policy gradient (DDPG) reinforcement learning model enables the optimal control of the SCR denitrification process in a power station.
Key words:multi-work condition; minibatchkmeans clustering; Stacking-XRLL; DDPG algorithm; optimal control
收稿日期:2021-12-07
基金项目:国家自然科学基金--广东省联合基金项目(U2001201);广东省基础与应用基础研究基金项目(2020B1515120010)。
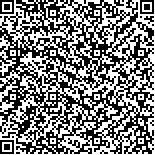