基于深度学习的航空发动机滑油磨粒检测研究
2022,30(4):14-22
摘要:针对滑油中磨粒形状复杂且尺寸大小不一,传统滑油磨粒检测方法存在时效性差、检测尺度小、精度低、非铁磁性磨粒不能检测等缺点。本文设计了一种基于深度学习的航空发动机滑油磨粒检测方法。基于连续流微流控芯片的滑油图像采样方法,构建滑油图像采样系统;设计图像增强方法,进行图像数据增强消融试验研究,针对YOLOv3模型和Faster RCNN模型进行精度测试,结果表明消融试验后的YOLOv3模型检测能力明显优于Faster RCNN模型;为减少消融后YOLOv3模型的误检率,提出SER算法以优化该模型的推理置信度阈值。研究结果表明滑油磨粒检测方法可解决传统测试中存在的问题,且在0.35的置信度阈值下, YOLOv3模型的检测结果能够达到94.2%的召回率和95.9%的精确度。
关键词:航空发动机;滑油磨粒检测;深度学习;YOLOv3模型;SER算法;
Research on Abrasive Detection of Aero-engie Lubricating Oil Based on Deep Learning
Abstract:In view of the complex shapes and different sizes of abrasive grains in lubricating oil, traditional lubricating oil abrasive grain detection methods have disadvantages such as poor timeliness, small detection scale, low accuracy, and non-ferromagnetic abrasive grains cannot be detected. The paper designs an aero-engine lubricating oil abrasive grain detection method based on deep learning. Based on the continuous flow microfluidic chip-based lubricant image sampling method, the lubricant image sampling system was constructed; The image enhancement method was designed, and the image data enhancement ablation experiment was carried out, the test accuracy of the YOLOv3 model and the Faster RCNN model was compared. The results show after the ablation test the detection ability of the YOLOv3 model is significantly better than the Faster RCNN model; in order to reduce the false detection rate of the YOLOv3 model after ablation, the SER algorithm is proposed to optimize the model’s inference confidence threshold. The research results show that the lubricating oil abrasive grain detection method can solve the problems in the traditional test, and under the confidence threshold of 0.35, the detection result of the YOLOv3 model can achieve a recall rate of 94.2% and an accuracy of 95.9%.
Key words:Aero engines; Lubricating oil abrasive detection; Deep learning; YOLOv3 Model; SER algorithm;
收稿日期:2021-09-24
基金项目:校级科研立项项目(2020KY0204)
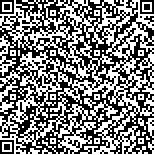