一种融合注意力机制与上下文信息的交通标志检测方法
2022,30(3):54-59
摘要:针对当前交通标志检测中存在小目标检测精度低、检测实时性不高以及目标漏检等问题,在YOLOv3基础上提出了一种融合了注意力机制与上下文信息的交通标志检测方法。首先通过改进通道注意力机制的压缩方式,对特征图通道重新进行标定;然后引入空间金字塔池化模块SPP;最后增加特征映射并拼接到原特征融合网络中的小目标部分,充分利用上下文信息增强对小目标的检测。实验结果表TT100K(Tsinghua-Tencent 100K)交通标志数据集上,与YOLOv3网络相比,在每秒传输帧数(Frame Per Second,FPS)变化不大的情况下,平均精度均值和小目标的精度均值分别提升3.03%和4.59%。实验结果证明了改进网络在小目标检测和整体检测中的有效性。
关键词:小目标检测;YOLOv3;注意力机制;SPP;上下文信息
A traffic sign detection based on attentional mechanism and contextual information
Abstract:A traffic sign detection method that combines attention mechanism and context information is proposed on the basis of YOLOv3, the method is proposed to address the problems of low accuracy of small targets, low real-time detection and missing target detection in current traffic sign detection. In this method, firstly, the channel of feature graph is re-calibrated by improving the compression method of channel attention mechanism, while the channel weight of less information is suppressed; then the spatial pyramid pooling module SPP is introduced to obtain multi-scale local information; finally, the feature mapping is added and spliced into the small target part of the original feature fu-sion network. The contextual information is fully used to enhance the detection of small targets. The experimental results show that on the TT100K (Tsinghua-Tencent 100K) traffic sign dataset, the improved network can detect targets more effectively compared with the origi-nal YOLOv3 network; with little change in frames per second (FPS), the average precision mean and the small target The average accuracy mean and small target mean were improved by 3.03% and 4.59%, respectively. The experimental results demonstrate the effectiveness of the improved network in small target detection and overall detection.
Key words:small target detection; YOLOv3; Attentional mechanism; SPP; contexts
收稿日期:2021-08-24
基金项目:陕西省科技计划重点项目(2017ZDCXL-GY-05-03)
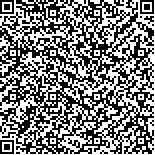